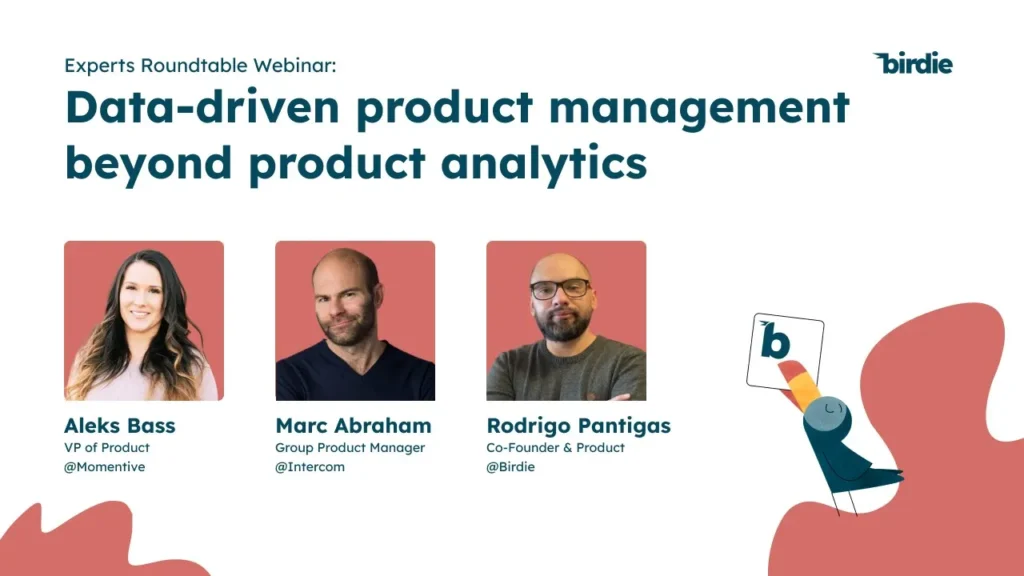
How Can Companies Apply Feedback Analysis Tools in Product Management to Better Understand Customer Needs?
No matter the industry, companies face an incredibly complex sales and marketing landscape, with consumers using multiple touchpoints to get informed, engage, and buy products – whether they are digital or physical products, B2B or B2C. They have one thing in common: the channels they turn to in order to purchase their products are mostly digital, and almost every consumer makes a decision before analyzing what other people are saying and interacting with a brand – as a reference, 94% of users today read reviews before deciding to buy.
As a consequence of that journey, data is being generated at an incredible speed and its importance is growing. Although getting access to what consumers are saying is not as hard anymore, finding the time to read, analyze, understand, and categorize that data is almost impossible – especially when companies try to do that with content from multiple data sources. That’s exactly where text analytics applied to customer opinions – or feedback analysis – comes in.
What is Feedback Analysis and why you should care about it?
Feedback Analysis is the application of Text Analytics to qualitative user feedback. Text Analytics is the process of interpreting and categorizing content, extracting context out of words. This process involves the automatic extraction and classification of written information according to relevant aspects of a specific situation. It can be applied to several different areas, from contracts to sales and marketing, and some of the traditional types of text analysis include sentiment analysis and keywords or topic detection.
In a Product Management context, Text Analytics can be applied to any qualitative user data, mostly user feedback, from consumer-generated content like support tickets, social comments, and conversations to data from user interviews and surveys. From there, a company can discover patterns that highlight behaviors and attributes or predict trends and use these insights in different stages of the Product Management Process, from Product Discovery to Delivery.
This type of analysis can be performed manually as long as a process is established, but it becomes extremely complicated to do it when larger subsets of data are included, especially from different structures and types. Taking the time to look at each text in detail and relating it to the context until finding patterns becomes inefficient and prone to errors.
Therefore, Text Analytics as it’s known today has become key – considering that 2.5 quintillion bytes are generated every day from multiple sources inside and outside organizations – to extract meaning from content in a scalable way, using text mining and NLP – Natural Language Processing to find context in huge amounts of text.
Companies who do not leverage Feedback Analysis in their Product Development processes will leave untapped data on the side, missing relevant insights not only about what their current customers think about them but also about how it is impacting other consumers. They will also miss the chance to discover what they could do differently to mitigate future problems and to potentialize the good aspects of the brand while reducing the impact of the bad aspects.
How to choose the right Feedback Analysis tool for Product Management – and get the best out of it
There are several Feedback Analysis tools and it is important to know how to differentiate one from another considering your use case. Many of the solutions available in the market are general-purpose tools that must be configured appropriately to give you the information and the granularity you want. So the first thing you should consider is what are your goals with Feedback Analysis and what specific insights and patterns you expect to find out – as its use can be very different depending if you are in Product Management, Marketing, or Customer Experience.
To keep the example within the Product Management space, let’s consider you want to structure data from consumer opinions (written in natural language) that come from different sources. There are some important things you need to know before choosing the correct tool, such as:
1. It’s out-of-the-box ability to extract metadata from the opinions
Extracting keywords out of conversations is the basic feature of a Text Analytics tool. Connecting each of these keywords to relevant business aspects that make sense to your company’s reality is a bit more complicated. Although some tools can still deliver some relevant metadata using queries and topics, there is still a lot of manual work left to do to relate them to your business. And that’s when having this ability out of the box can be crucial to save you time and ensure you get value out of the tool.
What is it about
What is your opinion about it? Is it the whole company, a specific product, a place, an experience, or a service? That is important because your analysis must be segmented enough to give you the ability to filter those opinions that are not your focus right now. Wrong segmentations might give you wrong information, conclusion, and actions.
If you want to know about aspects related to your product, for instance, granular user segmentation is important to you to know exactly which product version the consumer is given his/her opinion about. You might consider tools that enable you to extract and organize the information with granularity, ideally separating it according to different aspects – the product, the experience, and others.
Other important metadata:
Several other metadata can be used to segment, filter, classify, and finally better narrow your analysis. Think about your business needs and what kind of information you might consider. These are some ideas:
- The source type (support ticket, CRM, NPS, communities, social media, …);
- The date when the opinion was posted;
- The language in which it was written;
- The user segment and cohort;
- The geolocation (when available) of the consumer who posted the opinion;
- Other relevant metadata available from your sources.
2. It’s the ability to extract granular, structured details about the opinion
Most Feedback Analysis tools can extract important (frequent, trending, and so on) keywords and sentiments from the whole sentence. But this is just the very beginning. You might consider configuring the text analytics tools to a more granular level. Have a look at this way of doing it:
First, you must consider different types of domain-specific words (aspects) to be searched and extracted from the opinion, such as:
- Attributes (I love how the compartments of this refrigerator were designed.)
- Jobs (I was trying to upload a file to be able to move to the next step.)
- Personas (I bought this toy for my grandchildren but he does not like it.)
We generally call all of these domain-specific words ASPECTS of an opinion. You can then consider the traditional frequent, trending, and other measures over the aspects to have a better understanding of the opinion, eliminating most of the trash words that come with the general keyword approach.
Second, you should analyze sentiment on an aspect level against whole sentence sentiment. Well, you know in a granular way what the consumer is talking about with the aspect approach. But what judgment they are doing about each extract aspect specifically. If you want such a granular level, you need a tool that comes with an advanced level of aspect-based sentiment analysis. With this ability, for the examples above, the tool should be able to predict the following sentiments for each aspect:
- compartments = positive
- removing cat hair = positive
- grandchildren = negative
Third – and you can ignore this part if you want just to analyze a dozen specific aspects, but most of the time that is not the case. A unique product category might have thousands of different aspects that were evaluated by the consumers. Even though they are much better than the traditional keyword approach, there might be still too much information for a human to analyze.
Organizing these aspects in an explorable way is an important task to make a faster and effective analysis for your business. We suggest that you build a domain-specific taxonomy to organize all the fine-grain aspects and their sentiments. The taxonomy must group synthetic different (but with the same/similar meaning) words. For example cost, price, pricing, cheap, expensive – all of them are talking about price.
A taxonomy is a hierarchical way of organizing things, so you could also merge different groups of aspects and have, in the end, just a dozen high-level groups to analyze that you ideally can deep dive into detail whenever you want.
As you can probably tell by now, good Feedback Analysis is all about the details and getting specific. That’s the reason why we built a complex process with a high-quality and granular analysis that ensures nothing is wrongly interpreted. Birdie has also chosen to focus on Product Feedback Analysis, which means that our solution applies Text Analytics to Product Management, Product Marketing, and Customer Experience – all related to the product, which allows us to connect content to meaning and reduce the effort from our clients. Finally, Birdie has created an easy-to-use dashboard that makes insights easier to access and activate, ensuring our clients can optimize their time and prove the ROI of Consumer Insights.
Curious to see it in action? Join our waitlist.
Feedback Analytics Platform for a better product strategy
Birdie helps product-centric companies better understand customers at scale to create product strategies to increase acquisition, conversion, and retention.
Related Posts
4 Steps to Leverage Qualitative Data to Improve Customer Satisfaction and Reduce Support Cost
After multiple iterations of our own methodology to help our customers increase their customer satisfaction [...]
Leveraging Customer Feedback Analytics to Enhance Customer Experience in FinTech
Leveraging Customer Feedback Analytics to Enhance Customer Experience [...]
Navigating the Path to SaaS Success: Insights from Henrique at Bill.com
In the fast-paced world of Software as a Service (SaaS), success isn’t just about building [...]
Product Leader’s First 180 Days: Insights from Esteban’s Masterclass on Scaling Product and Teams
In the ever-evolving landscape of product development, effective leadership can make all the difference between [...]
Leveraging AI and Behavioral Economics for Community Building and User Engagement
Today it’s really important for businesses to create active online communities and get users involved. [...]
From Product Management to the C-Suite: Navigating Your Path to Leadership
The journey from a product management role to a leadership position in the C-suite is [...]
Building and Scaling Feedback Loops for Effective Product Development
In the dynamic world of product development, feedback is the lifeblood that fuels innovation and [...]
Successful Product Discovery: 4 Key Insights from a Sr. Product Manager at Miro
Successful Product Discovery [...]